Main navigation
This year's lecture
Speaker: Dr. Daniela Witten
Professor of Statistics and Biostatistics, and the Dorothy Gilford Endowed Chair in Mathematical Statistics, University of Washington
When: April 24 and April 25, 2024
Learn more about the speaker
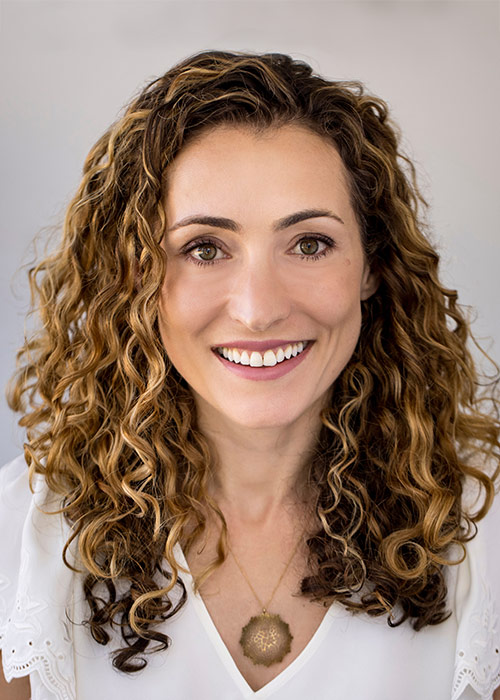
Daniela Witten is a professor of Statistics and Biostatistics at University of Washington, and the Dorothy Gilford Endowed Chair in Mathematical Statistics. She develops statistical machine learning methods for high-dimensional data, with a focus on unsupervised learning.
Daniela is the recipient of an NIH Director's Early Independence Award, a Sloan Research Fellowship, an NSF CAREER Award, and a Simons Investigator Award in Mathematical Modeling of Living Systems. She received the Presidents’ Award from the Committee of Presidents of Statistical Societies (COPSS), awarded annually to a statistician under age 41 in recognition of outstanding contributions to the field of statistics. She also received the Spiegelman Award from the American Public Health Association for a statistician under age 40 who has made outstanding contributions to statistics for public health, and the Leo Breiman Award for contributions to the field of statistical machine learning. She is a Fellow of the American Statistical Association and the Institute for Mathematical Statistics, and an Elected Member of the International Statistical Institute.
Daniela is a co-author (with Gareth James, Trevor Hastie, and Rob Tibshirani) of the very popular textbook Introduction to Statistical Learning. She has served as an Associate Editor for Biometrika, Journal of Computational and Graphical Statistics, and Journal of the American Statistical Association, and as an Action Editor for Journal of Machine Learning Research. Since 2023, she serves as Joint Editor of Journal of the Royal Statistical Society, Series B.
Daniela completed a BS in Math and Biology with Honors and Distinction at Stanford University in 2005, and a PhD in Statistics at Stanford University in 2010.
Schedule
Thursday, April 24
- 10:00 a.m. Refreshments and Awards in 302 SH
- 3:30 p.m. Lecture #1, LR2 VAN
"Selective Inference for Real-World Problems"
Abstract: Statistics textbooks typically assume that the data analyst has chosen a hypothesis to test or a confidence interval to estimate before looking at the data — or, better yet, before they have even collected it! However, in reality, statistical practice often proceeds quite differently: An analyst may first explore the data in order to come up with a statistical question that seems “interesting” and then use the same data to answer that question. This practice is often described as “double dipping.” Unfortunately, classical statistical machinery does not apply when we have double dipped: For instance, hypothesis tests will reject the null hypothesis far more often than they should, and confidence intervals will not cover the parameter of interest. This leads to spurious findings that will not hold up in future studies. In this talk, I will discuss recent developments that enable valid inference with double dipping. I will present the conditional selective inference framework as well as sample splitting and its variants. These ideas will be motivated using an application to single-cell RNA sequencing.
Friday, April 25
- 9:30 a.m. Lecture #2, W151 PBB
"Inference on a Single Realization of a Network"
Abstract: Given a dataset consisting of a single realization of a network, we consider conducting inference on a parameter selected from the data. For instance, suppose that we perform community detection to identify sets of nodes with generally similar connectivity patterns. Inference on the connectivity within and between the estimated communities poses a challenge, since the communities are themselves estimated from the data. Furthermore, since only a single realization of the network is available, sample splitting is not possible. In this talk, I will show that it is possible to split a single realization of a network consisting of n nodes into two (or more) networks involving the same n nodes; the first network can then be used to select a data-driven parameter, and the second to conduct inference on that parameter. In the case of weighted networks with Poisson or Gaussian edges, we obtain two independent realizations of the network; by contrast, in the case of Bernoulli edges, the realizations are dependent, and so extra care is required. We establish the theoretical properties of our estimators, in the sense of confidence intervals that attain the nominal (selective) coverage, and demonstrate their utility in numerical simulations and in application to a dataset representing the relationships among members of a karate club. This work is a collaboration with Ethan Ancell (U Washington) and Dan Kessler (U North Carolina).
History of the lectures
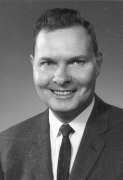
When the Department of Statistics and Actuarial Science was created in 1965, it had 5 faculty members: Bob Hogg, Allen Craig, John Birch, Lloyd Knowler, and Jim Hickman. Hogg was the founding chair of the department.
Craig, who earned his UI PhD in 1931, was the doctoral advisor of Hogg, who earned his UI PhD in 1950. By then, Craig had already made important contributions to the profession. Indeed, he was instrumental in getting the Institute of Mathematical Statistics started and was on the original (1938) editorial board of the IMS’s Annals of Mathematical Statistics, along with Jerzy Neyman and Sam Wilks (UI PhD 1931). Hogg would go on to make important contributions of his own, serving as program secretary for the IMS from 1968-1974 and president of the American Statistical Association in 1988.
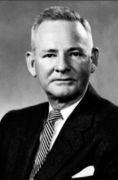
Hogg and Craig had different personalities but shared many of the same passions. They both loved statistics and they both were terrific scholars and educators. They teamed up in 1958 to write one of the most popular mathematical statistics books ever written–the book known eponymously as “Hogg and Craig.”
The annual Craig Lectures began when Allen Craig delivered his retirement talk in 1970. In the following years, Craig Lectures included Fred Mosteller, Brad Efron, Bob Hogg, Jim Hickman, Carl Morris, Herman Chernoff, Luke Tierney, and Alan Agresti. When Bob Hogg passed away in 2014, the lectures were renamed the Hogg and Craig Lectures. Past Hogg and Craig Lecturers include Dick Dykstra, Xiao-Li Meng, David Donoho, and Donald Rubin.
Historical timeline
Lecture 51
April 18-19, 2024Speaker: David Dunson, Duke University
Topics: "Improving understanding of life on earth through novel data and statistics" and "Novel models and algorithms for massive-dimensional and sparse multivariate data"Lecture 50
April 28 and 29, 2023Speaker: Dan Nettleton, Iowa State University
Topics: "My Adventures in Sports Statistics, Beginning With Bob Hogg" and "Who Is Winning? Determining Whether a Candidate Leads in a Ranked-Choice Election"Lecture 49
April 21 and 22, 2022Speaker: Donald B. Rubin, Harvard University
Topics: "Essential Concepts of Causal Inference: A Remarkable History and an Intriguing Future” and “Conditional Calibration and the Sage Statistician”Lecture 48
April 15 and 16, 2021Speaker: Bin Yu, University of California at Berkeley
Topics: "Veridical Data Science: the practice of responsible data analysis and decision-making” and “Iterative Random Forests (iRF) with applications to biomedical problems through epiTree for epistasis discovery”Lecture 47
April 25 and 26, 2019Speaker: David A. Harville, Iowa State University
Topics: "Ranking/Rating Basketball or Football Teams: the NCAA Way and the ‘Right’ Way” and “Model-Based Prediction in General and in the Special Case of Ordinal Data”Lecture 46
April 26 and 27, 2018Speaker: David Donoho, Stanford University
Topics: "50 Years of Data Science” and “Covariance Estimation in Light of the Spiked Covariance Model”Lecture 45
March 29 and 30, 2017Speaker: Xiao-Li Meng, Harvard University
Topics: "From Euler to Clinton: An Unexpected Statistical Journey (Or: Size Does Matter, but You Might be in for a Surprise…)” and “Bayesian, Fiducial, and Frequentist (BFF): Best Friends Forever?”Lecture 44
2015Speaker: Richard L. Dykstra, University of Iowa
Topics: "Fifty Years of Statistical Memories" and "Von Neumann's Alternating Projections and Dykstra's Algorithm"Semi-Centennial Symposium
2015We celebrated our department's Semi-Centennial Symposium this year!
Lectures renamed
2014The annual Craig Lectures are officially renamed to be the Hogg and Craig Lectures after Bob Hogg passes away.
Lecture 43
2014Speaker: Jianqing Fan, Princeton University
Topics: "Statistical Challenges in Analysis of Big Data" and "Homogeneity Pursuit"Lecture 42
2013Speaker: Paul Embrechts, ETH Zurich
Topics: “Thinking about Extremes” and “Model Uncertainty and Risk Aggregation”Lecture 41
2012Speaker: Rob Tibshirani, Stanford University
Topics: “Finding consistent patterns: A nonparametric approach for identifying differential expression in RNA-Seq data” and “The lasso: some novel algorithms and applications”Lecture 40
2011Speaker: Alan Gelfand, Duke University
Topics: "Space is the Place: Why spatial thinking matters for environmental problems" and "Point pattern modeling for degraded presence-only data over large regions"Lecture 39
2010Speaker: Terry Speed, University of California at Berkley
Topic: "Removing Unwanted Variation From Microarray Data and Analysis of ChIP-Seq Data"Lecture 38
2009Speaker: George Casella, University of Florida
Topics: "Estimation in Dirichlet Random Effects Models" and "From R. A. Fisher to Microarrays: Why 70-Year-Old Theory is Relevant Today"Lecture 37
2007Speaker: Nancy Reid, University of Toronto
Topics: "Weighting the Likelihood Function" and "Putting Asymptotics to Work"Lecture 36
2006Speaker: Alan Agresti, University of Florida
Topics: "Reducing Conservatism of Exact Small-Sample Inference for Discrete Data" and "A Twentieth Century Tour of Categorical Data Analysis"Lecture 35
2005Speaker: Jay Kadane, Carnegie Mellon University
Topics: "Driving While Black: Differential Enforcement of the Traffic Laws on the New Jersey Turnpike" and "Is Ignorance Bliss?"Lecture 34
2004Speaker: Jim Berger, Duke University
Topics: "Objective Bayesian Analysis: Its Uses in Practice and Its Role in the Unification of Statistics" and "Validation of Computer Models"Lecture 33
2003Speaker: Elizabeth Thompson, University of Washington
Topics: "Linkage Detection for Complex Traits" and "Monte Carlo Estimation of Likelihood Functions: The Example of Multipoint Linkage LOD Scores"Lecture 32
2001Speaker: Luke Tierney, University of Minnesota-Twin Cities
Topics: "Some Adaptive Monte Carlo Methods for Bayesian Inference" and "Some Issues in the Design of R"Lecture 31
2000Speaker: Hans Gerber, University of Lausanne (Switzerland)
Topics: "Trees R Us: From Kronecker and Esscher to Black and Scholes" and "Pricing Perpetual Options for Jump Processes: From Risk Theory to Finance"Lecture 30
1999Speaker: Howell Tong, London School of Economics and University of Hong Kong
Topics: "Chaos in Statistics" and "Some Recent Non-Parametric Tools in Nonlinear Time Series"Lecture 29
1998Speaker: Ulf Grenander, Brown University
Topics: "Computational Anatomy" and "A Bayesian Approach to Vision"Lecture 28
1997Speaker: John A. Hartigan, Yale University
Topics: "The Effect of Proposition 48 on Graduation Rates of American Athletes" and "The Maximum Likelihood Prior"Lecture 27
1996Speaker: Trevor Hastie, Stanford University
Topics: "Flexible Discriminant and Mixture Models" and "Metrics and Models for Handwritten Digit Recognition"Lecture 26
1995Speaker: F.T. (Tim) Wright, University of Missouri-Columbia
Topics: "Harnessing Chance" and "Pseudo Likelihood Inferences for Ordered Survival Curves Under the Assumption of Proportional Hazards"Lecture 25
1994Speaker: Peter McCullagh, University of Chicago
Topics: "The Role of Models in Statistics" and "Some Remarks on Over-Dispersion"Lecture 24
1993Speaker: Herman Chernoff, Harvard University
Topics: "An Application of a Result of Elfving on the Optimal Design of Regression Experiments" and "The Distribution of the Likelihood-Ratio for Mixtures of Distributions with Application to Genetics"Lecture 23
1992Speaker: Herbert Robbins, Columbia University
Topics: "Big N, Little n: Minimizing the Ethical Cost of a Clinical Trial" and "Estimation Under Biased Allocation"Lecture 22
1991Speaker: T.W. Anderson, Stanford University
Topics: "R.A. Fisher and Multivariate Analysis" and "Goodness-of-fit Tests for Spectral Distributions"Lecture 21
1990Speaker: Thomas P. Hettmansperger, Pennsylvania State University
Topics: "Simple Sign Based Inference in the Location Model" and "Rank Based Inference in the Linear Model".Lecture 20
1989Speaker: Ron Pyke, University of Washington
Topics: "The Bell-Shaped Curve: A Central Role for Probability in Statistics" and "Set-Indexed Empirical, Quantile and Rank Processes"Lecture 19
1988Speaker: Tom Ferguson, University of California-Berkeley
Topics: "Who Solved the Secretary Problem?" and "Some Time-Invariant Stopping Rule Problems"Lecture 18
1987Speaker: Carl Morris, University of Texas
Topics: "Parametric Empirical Bayes: An Overview" and "Bayesian Empirical Bayes Interval Estimation: A Review of Recent Progress"Lecture 17
1986Speaker: Steve Stigler, University of Wisconsin
Topics: "John Craig and the Probability of History" and "The History of Statistics in the Social Science: Recovering from the Central Limit Disaster"Lecture 16
1985Speaker: George E.P. Box, University of Wisconsin
Topics: "Analyzing Fractional Designs" and "Thoughts on Some Ideas of Genichi Taguchi"Lecture 15
1984Speaker: Wayne Fuller, Iowa State University
Topics: "Measurement Error in Regression" and "Nonlinear Measurement Error Models"Lecture 14
1983Speaker: J. Stuart Hunter, Princeton University
Topics: "Theory Sigma: Quality Through Statistical Methods" and "Fractional Factorials: Sequential and Prior Analysis"Lecture 13
1982Speaker: Colin L. Mallows, Bell Telephone Laboratories
Topics: "Robust Methods -- Applications and Basic Concepts" and "Robust Methods: Theory"Lecture 12
1981Speaker: David J. Bartholomew, London School of Economics and Political Science
Topic: "Latent Variable Models in Statistics"Lecture 11
1980Speaker: James C. Hickman, University of Wisconsin
Topics: "The Great Rates of Retirement Planning: Wages, Interest and Population" and "Bayesian Bivariate Graduation and Forecasting"Lecture 10
1979Speaker: Robert V. Hogg, University of Iowa
Topics: "On Statistics at Iowa: Before 1950" and "On Statistics at Iowa: After 1950"Lectures renamed
1978The annual lectures are officially renamed to be the Allen T. Craig Lecture Series after Professor Craig passes away.
Lecture 9
1978Speaker: J.L. Doob, University of Illinois
Topics: "A Discrete Boundary Value Problem" and "A General First Boundary Value Problem for Laplace's Equation"Lecture 8
1977Speaker: Frank Proschan, Florida State University
Topics: "A Class of Multivariate Functions in Ranking Problems" and "A Case History: Explaining an Observed Decreasing Failure Rate"Lecture 7
1976Speaker: Brad Efron, Stanford University
Topics: "How Many Words Did Shakespeare Know?" and "Regression and ANOVA with 0-1 Data"Lecture 6
1975Speaker: Dennis V. Lindley, University College, London
Topics: "Getting Married and Related Problems" and "Analysis of Variance"Lecture 5
1974Speaker: Jack Kiefer, Cornell University
Topics: "Foundations of Statistics: Are There Any?" and "How to Find an Optimum Design"Lecture 4
1973Speaker: H.D. Brunk, Oregon State University
Topics: "Bayesian Inference: Some Introductory Illustrations" and "Some Bayesian Approaches to Nonparametric Estimation"Lecture 3
1972Speaker: William Kruskal, University of Chicago
Topics: "Federal Statistics: People and Problems" and "Statistics: Public Policy and Private Understanding"Lecture 2
1971Speaker: Frederick Mosteller, Harvard University
Topic: "Statistics in Society"Lecture 1
1970Speaker: Allen T. Craig, University of Iowa
Topic: "Retirement Talk"