Main navigation
This year's lecture
Speaker: Dr. David Dunson
Arts and Sciences Distinguished Professor of Statistical Science
Trinity College of Arts & Sciences, Duke University
When: April 18 and April 19, 2024
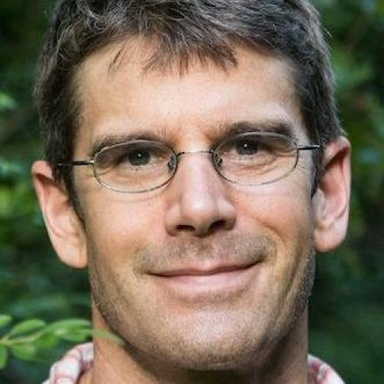
David Dunson's research focuses on developing statistical and machine learning methodology for analysis and interpretation of complex and high-dimensional data, with a particular emphasis on scientific applications, Bayesian statistics, and probability modeling approaches. Methods development and theory is directly motivated by challenging applications in neuroscience, genomics, environmental health, and ecology among others.
His work has had a substantial impact, with an H-index of 94. He has received numerous awards, including a gold medal from the U.S. Environmental Protection Agency, the COPSS Presidents' Award given to one outstanding statistician each year, the Mortimer Spiegelman Award given to one outstanding public health statistician each year, a highly cited researcher award from Web of Science, an IMS Medallion lecture, and most recently the G.W. Snedecor Award of the Committee of the Presidents of Statistical Societies (COPSS).
Dr. Dunson is a highly regarded researcher with a profound expertise in mathematics and statistics, particularly in the realm of machine learning. His research interests are centered around statistical science and its practical applications, where he is notably passionate about Bayesian modeling, computational statistics, and machine learning techniques. One of the key areas of his focus lies in developing cutting-edge methodologies to address challenges posed by complex and high-dimensional data in diverse fields, including epidemiology, neurosciences, and ecology.
In epidemiology, Dr. Dunson leverages machine learning algorithms to analyze large-scale health datasets, enabling a deeper understanding of disease transmission and risk factors. In neurosciences, he employs sophisticated machine learning approaches to glean insights from brain imaging data, unraveling the complexities of brain function and neurological disorders. Moreover, Dr. Dunson's contributions in ecology involve using machine learning to investigate intricate ecological patterns and dynamics, aiding conservation efforts and ecological management. Through his interdisciplinary and machine learning-driven approach, Dr. Dunson continues to push the boundaries of statistical science, leaving a lasting impact on various scientific disciplines.
Thursday, April 18
- 1:30 p.m. Refreshments and Awards in 302 Schaeffer Hall (SH)
- 3:30 p.m. Lecture #1 in 107 English-Philosophy Building (EPB)
Improving understanding of life on earth through novel data and statistics
Biodiversity data tend to be extremely biased towards large and charismatic organisms that are relatively easy to observe and accessible to human observers. We seek to address this gap and fundamentally improve understanding of life on earth through (relatively) unbiased automated monitoring of insects, fungi, birds and mammals at sites across the earth. Each site contains audio monitors (to identify bird vocalizations), camera traps (to detect mammals and large birds), malaise traps (to capture insects) and cyclone samplers (to capture fungal spores). Taxonomic classification of the insect and fungi species is based on DNA barcoding applied to the collected samples. There is interest in applying joint species distribution modeling (JSDMs) to infer the impact of covariates (habitat, environmental disruption, climate, etc.) on the biological communities being monitored, while also inferring interaction networks among the species. In addition, there is interest in the discovery of new species and the study of factors related to biodiversity. Our data contain large numbers of insects and fungi species that were previously unknown to science, and a fundamental aspect of the data is that most of the species being sampled are extremely rare. This talk will introduce our ERC-funded Lifeplan study and describe some of the exciting data being collected, while highlighting the important role of novel AI, machine learning and statistical methods in analyzing and interpreting the data.
Friday, April 19
- 2:30 p.m. Reception in 241 Schaeffer Hall (SH)
- 3:30 p.m. Lecture #2 in 107 English-Philosophy Building (EPB)
Novel models and algorithms for massive-dimensional and sparse multivariate data
Motivated by biodiversity data collected in our ongoing Lifeplan study, we seek to address fundamental challenges that arise in ecological joint species distribution modeling (JSDMs). Current JSDMs take the form of multivariate binary hierarchical regression models, with the binary outcome vector indicating occurrences of different species in a sample and covariates including factors such as habitat, environmental disruption, climate, etc. The state-of-the-art Hierarchical Modeling of Species Communities (HMSC) framework, which is broadly used in the ecology community, uses multivariate probit hierarchical factor regression models implemented in a Bayesian framework with Gibbs sampling. We are motivated by several challenges that arise in applying HMSC to fungi and insect data in Lifeplan and related studies: (1) current algorithms are too slow to handle all the 10,000s of species observed in the data and hence analyses have focused on common species; (2) statistical models are not structured to handle extremely rare species that are only observed a few times in the dataset; (3) in practice, we cannot pre-specify the species identities before collecting the data and indeed we discover species unknown to science as we sample. We propose several novel methods to address these problems, which are of broad independent interest in efficiently fitting factor and latent feature models to massive-dimensional data. We illustrate these methods through applications to several biodiversity datasets.
History of the lectures
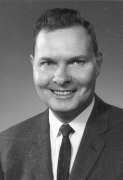
When the Department of Statistics and Actuarial Science was created in 1965, it had 5 faculty members: Bob Hogg, Allen Craig, John Birch, Lloyd Knowler, and Jim Hickman. Hogg was the founding chair of the department.
Craig, who earned his UI PhD in 1931, was the doctoral advisor of Hogg, who earned his UI PhD in 1950. By then, Craig had already made important contributions to the profession. Indeed, he was instrumental in getting the Institute of Mathematical Statistics started and was on the original (1938) editorial board of the IMS’s Annals of Mathematical Statistics, along with Jerzy Neyman and Sam Wilks (UI PhD 1931). Hogg would go on to make important contributions of his own, serving as program secretary for the IMS from 1968-1974 and president of the American Statistical Association in 1988.
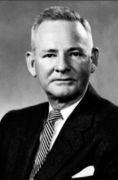
Hogg and Craig had different personalities but shared many of the same passions. They both loved statistics and they both were terrific scholars and educators. They teamed up in 1958 to write one of the most popular mathematical statistics books ever written–the book known eponymously as “Hogg and Craig.”
The annual Craig Lectures began when Allen Craig delivered his retirement talk in 1970. In the following years, Craig Lectures included Fred Mosteller, Brad Efron, Bob Hogg, Jim Hickman, Carl Morris, Herman Chernoff, Luke Tierney, and Alan Agresti. When Bob Hogg passed away in 2014, the lectures were renamed the Hogg and Craig Lectures. Past Hogg and Craig Lecturers include Dick Dykstra, Xiao-Li Meng, David Donoho, and Donald Rubin.
Historical timeline
Lecture 51
April 18-19, 2024Speaker: David Dunson, Duke University
Topics: "Improving understanding of life on earth through novel data and statistics" and "Novel models and algorithms for massive-dimensional and sparse multivariate data"Lecture 50
April 28 and 29, 2023Speaker: Dan Nettleton, Iowa State University
Topics: "My Adventures in Sports Statistics, Beginning With Bob Hogg" and "Who Is Winning? Determining Whether a Candidate Leads in a Ranked-Choice Election"Lecture 49
April 21 and 22, 2022Speaker: Donald B. Rubin, Harvard University
Topics: "Essential Concepts of Causal Inference: A Remarkable History and an Intriguing Future” and “Conditional Calibration and the Sage Statistician”Lecture 48
April 15 and 16, 2021Speaker: Bin Yu, University of California at Berkeley
Topics: "Veridical Data Science: the practice of responsible data analysis and decision-making” and “Iterative Random Forests (iRF) with applications to biomedical problems through epiTree for epistasis discovery”Lecture 47
April 25 and 26, 2019Speaker: David A. Harville, Iowa State University
Topics: "Ranking/Rating Basketball or Football Teams: the NCAA Way and the ‘Right’ Way” and “Model-Based Prediction in General and in the Special Case of Ordinal Data”Lecture 46
April 26 and 27, 2018Speaker: David Donoho, Stanford University
Topics: "50 Years of Data Science” and “Covariance Estimation in Light of the Spiked Covariance Model”Lecture 45
March 29 and 30, 2017Speaker: Xiao-Li Meng, Harvard University
Topics: "From Euler to Clinton: An Unexpected Statistical Journey (Or: Size Does Matter, but You Might be in for a Surprise…)” and “Bayesian, Fiducial, and Frequentist (BFF): Best Friends Forever?”Lecture 44
2015Speaker: Richard L. Dykstra, University of Iowa
Topics: "Fifty Years of Statistical Memories" and "Von Neumann's Alternating Projections and Dykstra's Algorithm"Semi-Centennial Symposium
2015We celebrated our department's Semi-Centennial Symposium this year!
Lectures renamed
2014The annual Craig Lectures are officially renamed to be the Hogg and Craig Lectures after Bob Hogg passes away.
Lecture 43
2014Speaker: Jianqing Fan, Princeton University
Topics: "Statistical Challenges in Analysis of Big Data" and "Homogeneity Pursuit"Lecture 42
2013Speaker: Paul Embrechts, ETH Zurich
Topics: “Thinking about Extremes” and “Model Uncertainty and Risk Aggregation”Lecture 41
2012Speaker: Rob Tibshirani, Stanford University
Topics: “Finding consistent patterns: A nonparametric approach for identifying differential expression in RNA-Seq data” and “The lasso: some novel algorithms and applications”Lecture 40
2011Speaker: Alan Gelfand, Duke University
Topics: "Space is the Place: Why spatial thinking matters for environmental problems" and "Point pattern modeling for degraded presence-only data over large regions"Lecture 39
2010Speaker: Terry Speed, University of California at Berkley
Topic: "Removing Unwanted Variation From Microarray Data and Analysis of ChIP-Seq Data"Lecture 38
2009Speaker: George Casella, University of Florida
Topics: "Estimation in Dirichlet Random Effects Models" and "From R. A. Fisher to Microarrays: Why 70-Year-Old Theory is Relevant Today"Lecture 37
2007Speaker: Nancy Reid, University of Toronto
Topics: "Weighting the Likelihood Function" and "Putting Asymptotics to Work"Lecture 36
2006Speaker: Alan Agresti, University of Florida
Topics: "Reducing Conservatism of Exact Small-Sample Inference for Discrete Data" and "A Twentieth Century Tour of Categorical Data Analysis"Lecture 35
2005Speaker: Jay Kadane, Carnegie Mellon University
Topics: "Driving While Black: Differential Enforcement of the Traffic Laws on the New Jersey Turnpike" and "Is Ignorance Bliss?"Lecture 34
2004Speaker: Jim Berger, Duke University
Topics: "Objective Bayesian Analysis: Its Uses in Practice and Its Role in the Unification of Statistics" and "Validation of Computer Models"Lecture 33
2003Speaker: Elizabeth Thompson, University of Washington
Topics: "Linkage Detection for Complex Traits" and "Monte Carlo Estimation of Likelihood Functions: The Example of Multipoint Linkage LOD Scores"Lecture 32
2001Speaker: Luke Tierney, University of Minnesota-Twin Cities
Topics: "Some Adaptive Monte Carlo Methods for Bayesian Inference" and "Some Issues in the Design of R"Lecture 31
2000Speaker: Hans Gerber, University of Lausanne (Switzerland)
Topics: "Trees R Us: From Kronecker and Esscher to Black and Scholes" and "Pricing Perpetual Options for Jump Processes: From Risk Theory to Finance"Lecture 30
1999Speaker: Howell Tong, London School of Economics and University of Hong Kong
Topics: "Chaos in Statistics" and "Some Recent Non-Parametric Tools in Nonlinear Time Series"Lecture 29
1998Speaker: Ulf Grenander, Brown University
Topics: "Computational Anatomy" and "A Bayesian Approach to Vision"Lecture 28
1997Speaker: John A. Hartigan, Yale University
Topics: "The Effect of Proposition 48 on Graduation Rates of American Athletes" and "The Maximum Likelihood Prior"Lecture 27
1996Speaker: Trevor Hastie, Stanford University
Topics: "Flexible Discriminant and Mixture Models" and "Metrics and Models for Handwritten Digit Recognition"Lecture 26
1995Speaker: F.T. (Tim) Wright, University of Missouri-Columbia
Topics: "Harnessing Chance" and "Pseudo Likelihood Inferences for Ordered Survival Curves Under the Assumption of Proportional Hazards"Lecture 25
1994Speaker: Peter McCullagh, University of Chicago
Topics: "The Role of Models in Statistics" and "Some Remarks on Over-Dispersion"Lecture 24
1993Speaker: Herman Chernoff, Harvard University
Topics: "An Application of a Result of Elfving on the Optimal Design of Regression Experiments" and "The Distribution of the Likelihood-Ratio for Mixtures of Distributions with Application to Genetics"Lecture 23
1992Speaker: Herbert Robbins, Columbia University
Topics: "Big N, Little n: Minimizing the Ethical Cost of a Clinical Trial" and "Estimation Under Biased Allocation"Lecture 22
1991Speaker: T.W. Anderson, Stanford University
Topics: "R.A. Fisher and Multivariate Analysis" and "Goodness-of-fit Tests for Spectral Distributions"Lecture 21
1990Speaker: Thomas P. Hettmansperger, Pennsylvania State University
Topics: "Simple Sign Based Inference in the Location Model" and "Rank Based Inference in the Linear Model".Lecture 20
1989Speaker: Ron Pyke, University of Washington
Topics: "The Bell-Shaped Curve: A Central Role for Probability in Statistics" and "Set-Indexed Empirical, Quantile and Rank Processes"Lecture 19
1988Speaker: Tom Ferguson, University of California-Berkeley
Topics: "Who Solved the Secretary Problem?" and "Some Time-Invariant Stopping Rule Problems"Lecture 18
1987Speaker: Carl Morris, University of Texas
Topics: "Parametric Empirical Bayes: An Overview" and "Bayesian Empirical Bayes Interval Estimation: A Review of Recent Progress"Lecture 17
1986Speaker: Steve Stigler, University of Wisconsin
Topics: "John Craig and the Probability of History" and "The History of Statistics in the Social Science: Recovering from the Central Limit Disaster"Lecture 16
1985Speaker: George E.P. Box, University of Wisconsin
Topics: "Analyzing Fractional Designs" and "Thoughts on Some Ideas of Genichi Taguchi"Lecture 15
1984Speaker: Wayne Fuller, Iowa State University
Topics: "Measurement Error in Regression" and "Nonlinear Measurement Error Models"Lecture 14
1983Speaker: J. Stuart Hunter, Princeton University
Topics: "Theory Sigma: Quality Through Statistical Methods" and "Fractional Factorials: Sequential and Prior Analysis"Lecture 13
1982Speaker: Colin L. Mallows, Bell Telephone Laboratories
Topics: "Robust Methods -- Applications and Basic Concepts" and "Robust Methods: Theory"Lecture 12
1981Speaker: David J. Bartholomew, London School of Economics and Political Science
Topic: "Latent Variable Models in Statistics"Lecture 11
1980Speaker: James C. Hickman, University of Wisconsin
Topics: "The Great Rates of Retirement Planning: Wages, Interest and Population" and "Bayesian Bivariate Graduation and Forecasting"Lecture 10
1979Speaker: Robert V. Hogg, University of Iowa
Topics: "On Statistics at Iowa: Before 1950" and "On Statistics at Iowa: After 1950"Lectures renamed
1978The annual lectures are officially renamed to be the Allen T. Craig Lecture Series after Professor Craig passes away.
Lecture 9
1978Speaker: J.L. Doob, University of Illinois
Topics: "A Discrete Boundary Value Problem" and "A General First Boundary Value Problem for Laplace's Equation"Lecture 8
1977Speaker: Frank Proschan, Florida State University
Topics: "A Class of Multivariate Functions in Ranking Problems" and "A Case History: Explaining an Observed Decreasing Failure Rate"Lecture 7
1976Speaker: Brad Efron, Stanford University
Topics: "How Many Words Did Shakespeare Know?" and "Regression and ANOVA with 0-1 Data"Lecture 6
1975Speaker: Dennis V. Lindley, University College, London
Topics: "Getting Married and Related Problems" and "Analysis of Variance"Lecture 5
1974Speaker: Jack Kiefer, Cornell University
Topics: "Foundations of Statistics: Are There Any?" and "How to Find an Optimum Design"Lecture 4
1973Speaker: H.D. Brunk, Oregon State University
Topics: "Bayesian Inference: Some Introductory Illustrations" and "Some Bayesian Approaches to Nonparametric Estimation"Lecture 3
1972Speaker: William Kruskal, University of Chicago
Topics: "Federal Statistics: People and Problems" and "Statistics: Public Policy and Private Understanding"Lecture 2
1971Speaker: Frederick Mosteller, Harvard University
Topic: "Statistics in Society"Lecture 1
1970Speaker: Allen T. Craig, University of Iowa
Topic: "Retirement Talk"