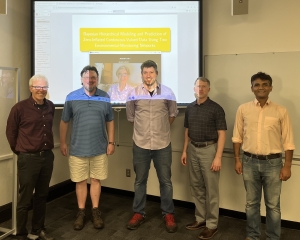
Congratulations Alex!
The National Weather Service (NWS) of the United States gathers and catalogues precipitation data at many sites across the country. The civilian-run complement for gathering precipitation data is known as the Community Collaborative Rain, Hail & Snow Network (CoCoRaHS). The CoCoRaHS network provides many more locations of precipitation data than the NWS; yet, CoCoRaHS having data come from a different environmental monitoring network may have different bias and variability compared to NWS. The two data sets are similar in the way that they both have zero inflation and atypical correlation structure due to the edge of precipitation storms. Using the data provided by CoCoRaHS and NWS in the mid-west region of the United States, we present a statistical analysis that has several main goals --- we determine the helpfulness of the citizen-based data. We propose a model for estimation and prediction of precipitation including the zero-inflated data in addition to determining the proportion of observed positive values that are necessary in order for model fitting to be effective. Furthermore, we accomplish all of these goals while developing computationally-efficient model fitting-strategies.
The data was analyzed in a Bayesian framework using a Gaussian Markov random field (GMRF) spatial model with a zero-inflated Tobit likelihood. After constructing a spatial model, a spatio-temporal model was created using an intrinsic Gaussian Markov random field (IGMRF). Finally, we conclude with a spatio-temporal model for monthly average rainfall that includes a seasonal precision structure. The metrics we used to assess “best” model was predictive coverage, credible set width, mean squared predictive error, efficient LOO-CV, and WAIC.
Committee: Kate Cowles and Sanvesh Srivastava (co-chairs)
Committee Members: Matt Bognar, Joseph Lang, Brian Smith (Biostatistics)