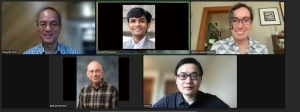
"Topics in Matrix Time Series Analysis"
This thesis research is motivated by the question of how to effectively detect a specific type of aurora known as pulsating aurora in video data captured by all-sky cameras. By considering each image as a matrix, a video can be represented as a sequence of matrices. These matrix sequences exhibit different patterns during different phases, such as phases with pulsating auroras and phases with no auroras. To capture and analyze this phenomenon, we propose a mixture autoregressive model for matrix-valued time series data. This model enables clustering of the sequences into distinct phases and allows for the study of dynamic patterns within each phase. We propose an EM algorithm for maximum likelihood estimation. We derive some theoretical properties of the proposed method including consistency and asymptotic distribution, and illustrate its performance via simulations and real applications.
Furthermore, we propose a parsimonious regression model for modeling matrix-valued data. This model assumes low-rank structures for the parameter matrices. We investigate the asymptotic properties of the least squares estimator and present a regularized approach for simultaneous parameter estimation and rank selection.
Given the vast amount of auroral data available, we also implement deep learning techniques, such as image and video classification methods, for aurora detection. Additionally, we introduce a spatiotemporal model for integrating the video data from several cameras across North America.
Committee Chair: Kung-Sik Chan
Committee Members: Allison Jaynes (Physics & Astronomy), Sanvesh Srivastava, Dale Zimmerman